Today, thanks in large part to the cloud, actions such as communicating over text or transferring funds digitally are so commonplace, we hardly even think about how incredible these processes are; as we enter the golden age of machine learning, we can expect a similar boom of benefits that previously seemed impossible.
Machine learning is already helping companies make better and faster decisions. In healthcare, the use of predictive models created with machine learning is accelerating research and discovery of new drugs and treatment regiments. In other industries, it’s helping remote villages of Southeast Africa gain access to financial services, and matching individuals experiencing homelessness with housing.
While the short term applications are encouraging, machine learning could potentially have an even greater impact on our society. In the future, machine learning will be intertwined and under the hood of almost every application, business process, and end-user experience. However, before this technology becomes so ubiquitous that it’s almost boring, there are four key barriers to adoption we need to clear first.
Democratizing machine learning
The only way that machine learning will truly scale is if we as an industry make it easier for everyone – regardless of their skill level or resources – to be able to incorporate this sophisticated technology into applications and business processes.
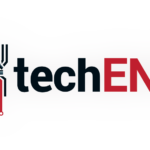
To achieve this, companies should take advantage of tools that have intelligence directly built into applications that their entire organization can benefit from. For instance, 123RF, a homegrown stock photography portal, aims to make design smarter, faster, and easier for users. To do so, it relies on Amazon Athena, Amazon Kinesis, and AWS Lambda for data pipeline processing. Its newer product Designs.ai Videomaker uses Amazon Polly to create voice-overs in more than 10 different languages. With AWS, 123RF has maintained flexibility in scaling its infrastructure and shortened product development cycles and is looking to incorporate other services to support its machine learning & AI research.
As processes go from being manual to automatic, workers are free to innovate and invent, and companies are empowered to be proactive instead of reactive. And as this technology becomes more intuitive and accessible, it can be applied to nearly every problem imaginable–from the toughest challenges in the IT department, to the biggest environmental issues in the world.
Upskilling workers
According to the World Economic Forum, the growth of AI could create 58 million net new jobs in the next few years. However, research suggests that there are currently only 300,000 AI engineers worldwide, and AI-related job postings are three times that of job searches with a widening divergence. Given this significant gap, organizations need to recognize that they simply aren’t going to be able to hire all the data scientists they need as they continue to implement machine learning into their work. Moreover, this pace of innovation will open doors and ultimately create jobs we can’t even begin to imagine today.
That’s why companies in the region like Asia Pacific University, DBS, Halodoc and others are finding innovative ways to encourage and nurture more young talents to gain new machine learning skills in fun, interactive hands-on ways, such as the AWS DeepRacer League. It’s critical that organizations should not only direct their efforts towards training the workforce they have with machine learning skills, but also invest in training programs that develop these important skills in the workforce of tomorrow.
Instilling trust in products
With anything new, often people are of two minds – either an emerging technology is a panacea and global savior, or it is a destructive force with cataclysmic tendencies. The reality is more often than not, a nuance somewhere in the middle. These disparate perspectives can be reconciled with information, transparency, and trust.
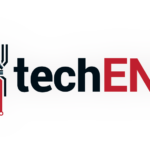
As a first step, leaders in the industry need to help companies and communities learn about machine learning, how it works, where it can be applied, ways to use it responsibly, and understand what it is not.
Second, in order to gain faith in machine learning products, they need to be built by diverse groups of people across gender, race, age, national origin, sexual orientation, disability, culture, and education. We will all benefit from individuals who bring varying backgrounds, ideas, and points of view to inventing new machine learning products.
Third, machine learning services should be rigorously tested, measuring accuracy against third party benchmarks. Benchmarks should be established by academia, as well as governments, and be applied to any machine learning-based service, creating a rubric for reliable results, as well as contextualizing results for use cases.
Regulation of machine learning
Finally, as a society, we need to agree on what parameters should be put in place governing how and when machine learning can be used. With any new technology, there has to be a balance in protecting civil rights while also allowing for continued innovation and practical application of the technology.
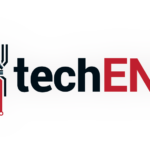
Any organization working with machine learning technology should be engaging customers, researchers, academics, and others to best determine the benefits of its machine learning technology with the potential risks. And they should be in active conversation with policymakers, supporting legislation, and creating their own guidelines for the responsible use of machine learning technology. Transparency, open dialogue, and constant evaluation must always be prioritized to ensure that machine learning is applied appropriately and is continuously enhanced.
What’s next
Through machine learning we’ve already accomplished so much, and yet, it’s still day one (and we haven’t even had a cup coffee yet!). If we’re using machine learning to help endangered orangutans, just imagine how it could be used to help save and preserve our oceans and marine life. If we’re using this technology to create digital snapshots of the planet’s forests in real-time, imagine how it could be used to predict and prevent forest fires. If machine learning can be used to help connect small-holder farmers to the people and resources they need to achieve their economic potential, imagine how it could help end world hunger.
To achieve this reality, we as an industry, have a lot of work ahead of us. I’m incredibly optimistic that machine learning will help us solve some of the world’s toughest challenges and create amazing end-user experiences we’ve never even dreamt. Before we know it, machine learning will be as familiar as reaching for our phones.